
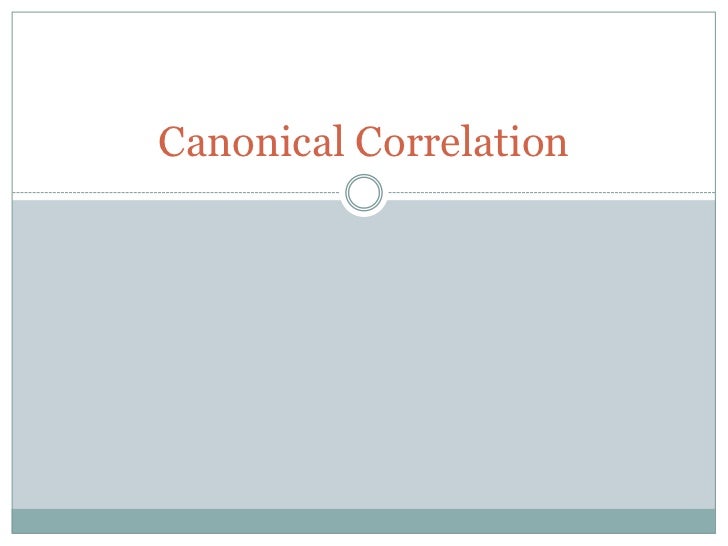
Compared with other state-of-the-art approaches, the proposed framework can achieve a comparable, or even better, performance in terms of global face reconstruction and the visual quality of face hallucination. In addition, in the residual-compensation process, a linear-mapping method is proposed to include both the inter- and intrainformation about manifolds of different resolutions. We propose using orthogonal CCA, which is proven by experiments to achieve a better performance in terms of global face reconstruction. The original CCA does not own the orthogonality property, which is crucial for information reconstruction. Canonical correlation analysis (CCA) with the orthogonality property is then employed, to maximize the correlation between the PCA coefficients of the LR and the HR face pairs to improve the hallucination performance. In the proposed algorithm, face images are first represented using principal component analysis (PCA). A two-step face-hallucination framework is proposed to reconstruct a high-resolution (HR) version of a face from an input low-resolution (LR) face, based on learning from LR–HR example face pairs using orthogonal canonical correlation analysis (orthogonal CCA) and linear mapping.
